The Ultimate Guide to Localization for AI Training Data: Go Global or Go Home
Ever noticed how your AI assistant nails it in English but totally fumbles when you throw in a phrase from your native tongue? Or how that chatbot you’re chatting with feels like it’s from another planet when it tries to understand your local slang? The problem isn’t the AI’s smarts, it’s the data it’s trained on. That’s localization for AI training data, the unsung hero making AI models globally savvy and culturally woke.
AI and machine learning are taking over the world, literally. From voice assistants to recommendation engines, these technologies are reshaping how we live, work, and even think.
But here’s the kicker: AI isn’t born smart. It learns from data, tons of it. And just like you can’t expect to ace a test without studying the right material, AI can’t perform well without high-quality training data. It’s the backbone of every smart system out there.
But here’s the catch: not all data is created equal. If you’re training an AI model to work globally, feeding it English-only datasets just won’t cut it. That’s where AI dataset localization comes in.
It’s all about adapting data to fit different languages, cultures, and contexts, think of it as teaching AI to speak the language of its users, whether that’s Spanish, Swahili, or even regional dialects.
Without language adaptation for machine learning, your AI might as well be shouting into the void. And let’s be real, nobody wants a monolingual AI in a multilingual world.
The future of AI isn’t just about being smart, it’s about being inclusive. Multilingual AI training data ensures that AI models don’t just understand words but also the nuances, idioms, and cultural quirks that make each language unique.
Because at the end of the day, an AI that gets you is an AI you’ll actually use. And that’s what localization is all about, making AI work for everyone, everywhere.
So, ready to dive into the world of localization for AI training data? Let’s break it down.
What is Localization in the Context of AI Training Data?
Alright, so we’ve established that localization for AI training data is the secret sauce behind making AI models globally friendly. But what exactly does that mean? Let’s break it down.
- Localization vs. Translation: Not the Same Thing
First off, localization isn’t just translation on steroids, it’s a whole different ball game. Translation is about converting words from one language to another. Localization, on the other hand, is about adapting content to fit a specific culture, region, or audience.
It’s the difference between saying “Hello” and saying “Howdy, y’all!” depending on whether you’re in New York or Texas. For AI, this means tweaking not just the language but also the context, tone, and even visuals to make sure it resonates with users.
- Why Localization is Critical for AI Training Data
Now, why does this matter for AI? Well, imagine training an AI model to understand English and then expecting it to flawlessly handle Japanese or Arabic. Spoiler alert: it won’t. That’s where AI dataset localization comes in.
For natural language processing (NLP) models, localization ensures the AI doesn’t just translate words but also understands idioms, slang, and cultural references. For computer vision models, it means teaching the AI to recognize objects, symbols, or gestures that might mean different things in different cultures.
Think about it: a thumbs-up might mean “good job” in the U.S., but it’s offensive in some parts of the Middle East. Without language adaptation for machine learning, your AI could end up making some seriously awkward mistakes. And let’s be real, nobody wants a chatbot that’s culturally clueless.
- Real-World Examples of Localized AI
So, where do we see this in action? Everywhere. Take voice assistants like Siri or Alexa. They’re not just multilingual, they’re localized. Siri knows how to respond to a British “Cheers, mate!” as well as an American “Thanks, dude!”
Chatbots in customer service? They’re trained on multilingual AI training data to handle queries in everything from French to Filipino. Even recommendation systems on platforms like Netflix or Spotify use localization to suggest shows or songs that vibe with your cultural preferences.
In short, localization isn’t just a nice-to-have, it’s a must-have if you want your AI to be truly global. And as we dive deeper into the world of AI, it’s clear that localization for AI training data is what separates the good from the groundbreaking.
Up next, let’s talk about why this stuff matters even more than you might think. Spoiler: it’s not just about avoiding awkward moments, it’s about building AI that works for everyone, everywhere.
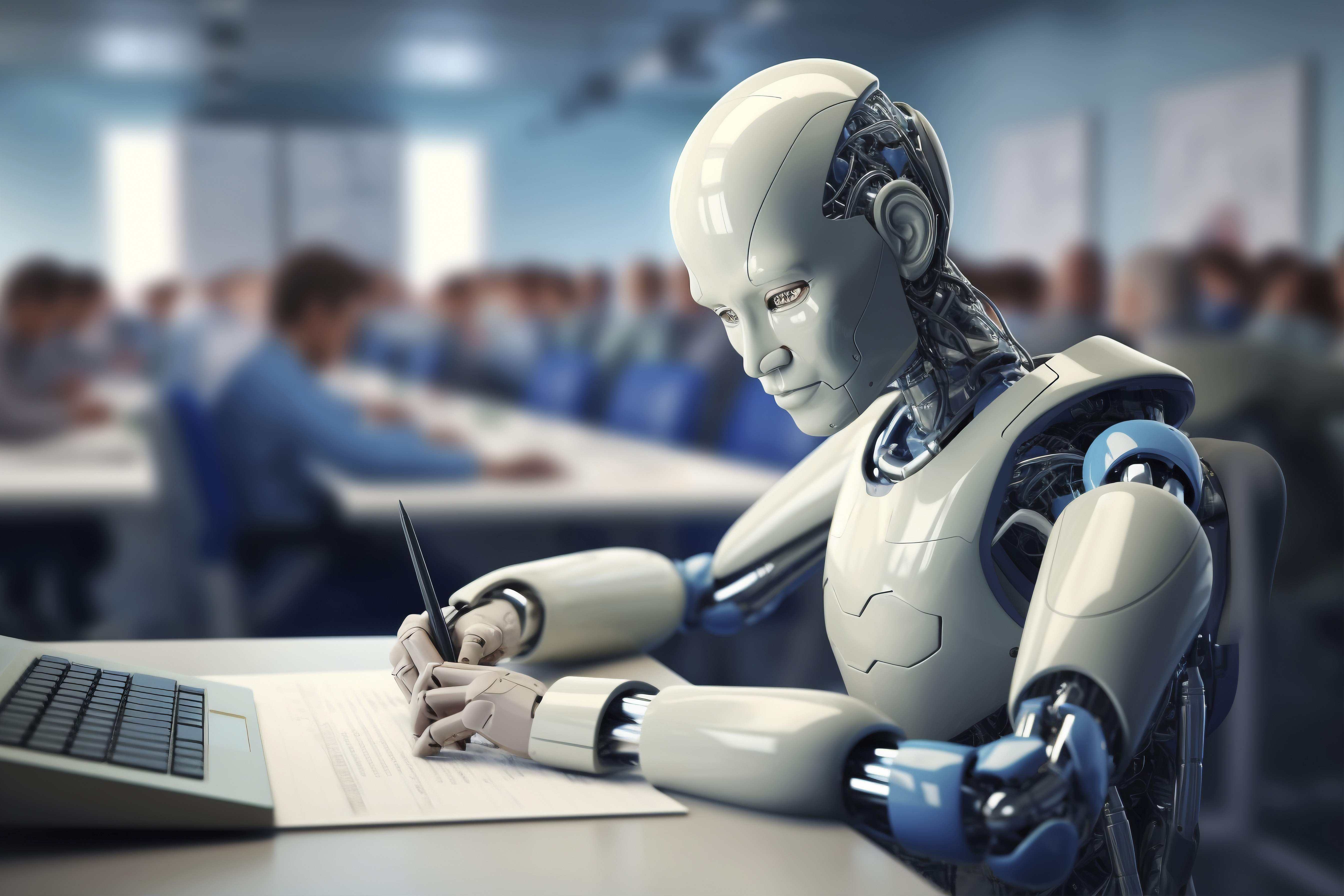
Why Should Businesses Care About Localization for AI Training Data?
Alright, so we’ve talked about what localization is and why it’s a big deal for AI. But let’s get real for a second: Why should businesses care? After all, isn’t it easier (and cheaper) to just stick with a one-size-fits-all, monolingual AI model? Spoiler: nope. Here’s why.
- The Pitfalls of Monolingual or Monocultural Datasets
Imagine this: You’ve built an AI model trained entirely on American English data. It works great until you try to use it in India, Japan, or Brazil. Suddenly, it’s struggling to understand accents, missing cultural references, or worse, offending users with tone-deaf responses.
That’s the problem with monolingual or monocultural datasets, they’re like trying to navigate a global city with a map of one neighborhood. You’re gonna get lost, and your users are gonna notice.
Without localization for AI training data, your AI is basically flying blind in any market that doesn’t match its training. And in today’s global economy, that’s a one-way ticket to irrelevance.
- How Localized Data Improves Accuracy, Inclusivity, and User Experience?
Here’s where AI dataset localization comes in clutch. By adapting your training data to different languages, cultures, and contexts, you’re not just making your AI smarter, you’re making it more inclusive. Think about it:
- Accuracy: A localized AI understands regional slang, dialects, and idioms. It doesn’t just translate, it gets you.
- Inclusivity: Multilingual AI training data ensures your AI works for everyone, not just English speakers. That’s a huge win for user trust and brand reputation.
- User Experience: When your AI feels like it’s from the user’s world, not some distant tech bubble, engagement goes through the roof.
For example, a chatbot that can switch seamlessly between Spanish and English, and understand the cultural nuances of both, is going to crush it in markets like the U.S. or Latin America. That’s the power of language adaptation for machine learning.
- The Risks of Ignoring Localization
But here’s the point: if you skip localization, you’re not just missing out on opportunities, you’re risking some serious backlash. AI models trained on narrow datasets can end up biased, culturally insensitive, or just plain wrong.
Ever heard of an AI mistaking a harmless gesture for something offensive? Or a voice assistant that can’t understand certain accents? Yeah, that’s what happens when localization isn’t a priority.
Worse, these mistakes don’t just frustrate users, they can damage your brand. In a world where consumers value inclusivity and cultural sensitivity, a tone-deaf AI is a PR disaster waiting to happen.
Localization isn’t just a technical detail, it’s a business imperative. Next up, let’s dive into the nitty-gritty of how to actually pull this off. Because let’s face it: AI dataset localization isn’t easy, but it’s totally worth it.
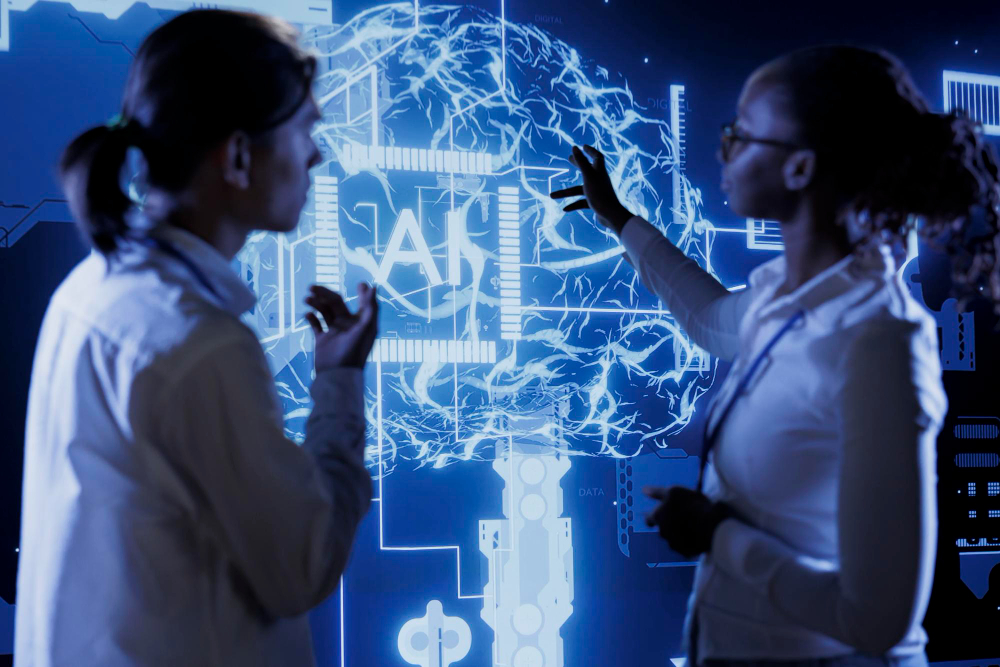
Key Challenges in Localizing AI Training Data
Alright, so we’ve established that localization for AI training data is a game-changer for businesses. But let’s not sugarcoat it: localization isn’t a walk in the park. It’s more like navigating a maze blindfolded, with a few hurdles along the way.
Here are the big challenges businesses face when diving into AI dataset localization and why they’re worth tackling head-on.
-
Capturing Cultural Nuances and Context
Let’s start with the obvious: culture is complicated. Sure, you can translate words, but can you capture the feel of a language? That’s where things get tricky. For example, sarcasm in English doesn’t always land the same way in Japanese. And don’t even get us started on regional slang or idioms.
Without nailing these nuances, your AI might end up sounding like a clueless tourist. Imagine a chatbot trying to crack a joke in German and accidentally offending someone instead. Yikes. That’s why language adaptation for machine learning isn’t just about words; it’s about context, tone, and cultural sensitivity.
-
Handling Low-Resource Languages
Here’s the thing: Not all languages are created equal in the AI world. While English, Spanish, and Mandarin have tons of data to work with, what about languages like Swahili, Basque, or Maori? These are what we call low-resource languages, they don’t have the same volume of digital content or datasets available.
Training AI models for these languages is like trying to bake a cake with half the ingredients. You’ve got to get creative, whether that means partnering with local communities, using transfer learning, or even creating synthetic data. It’s a challenge, but hey, inclusivity is worth the effort, right?
-
Ensuring Data Privacy and Compliance
Data is the lifeblood of AI, but it’s also a minefield when it comes to privacy and regulations. Different regions have different rules — GDPR in Europe, CCPA in California, you name it. And when you’re dealing with multilingual AI training data, you’ve got to make sure you’re not accidentally collecting or sharing sensitive information.
Oh, and let’s not forget about ethical concerns. Using data from certain communities without their consent? Big no-no. Businesses need to walk the tightrope between gathering enough data to train their models and respecting user privacy. It’s a balancing act, but one that’s non-negotiable.
-
Managing Cost and Scalability
Let’s talk money. Localization isn’t cheap. Hiring native speakers, cultural experts, and data annotators? That adds up. And if you’re targeting multiple languages and regions, the costs can skyrocket faster than a SpaceX launch.
Then, there’s scalability. Sure, you can localize for one or two languages, but what happens when you want to expand to 10 or 20? Suddenly, you’re dealing with a logistical nightmare. Businesses need to find ways to streamline the process, whether that’s through automation, crowdsourcing, or smart partnerships.
Why Are These Challenges Worth It?
Now, you might be thinking, “This sounds like a lot of work. Is it even worth it?” The short answer: Absolutely. Yes, localization for AI training data comes with its fair share of challenges, but the payoff is huge. We’re talking about AI models that are accurate, inclusive, and culturally aware models that don’t just work globally but appeal globally.
And let’s be real: in a world where consumers are increasingly demanding personalized, culturally sensitive experiences, skimping on localization isn’t an option. It’s a competitive advantage.
So, now that we’ve laid out the challenges, what’s the game plan? In the next section, we’ll dive into best practices for overcoming these hurdles and making AI dataset localization work for your business.
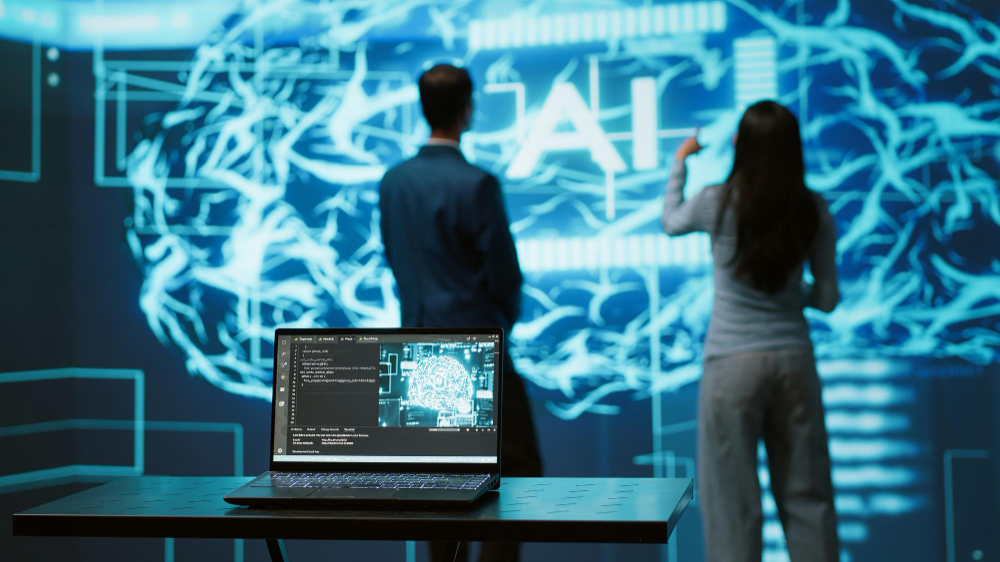
Best Practices for Localizing AI Training Data
Alright, so we’ve talked about the why and the what of localization for AI training data. Now, let’s get into the how. Because let’s face it: knowing the challenges is one thing, but actually pulling off effective AI dataset localization? That’s where the magic happens. Here are some actionable tips to help you nail it like a pro.
- Collaborate with Native Speakers and Cultural Experts
First rule of localization: don’t wing it. If you want your AI to sound like it’s from the region it’s serving, you need to bring in the experts. Native speakers and cultural consultants are your secret weapon. They’ll catch the nuances, slang, and context that even the fanciest algorithms might miss.
Think of it like this: you wouldn’t ask someone who’s never been to New York to write a guidebook for the city, right? Same logic applies here. Whether it’s French from France vs. Canadian French or Spanish from Spain vs. Latin America, the devil’s in the details.
- Use Region-Specific Datasets and Diversify Sources
Not all data is created equal. If you’re training your AI on generic, one-size-fits-all datasets, you’re setting yourself up for failure. Instead, go for region-specific datasets that reflect the language, culture, and context of your target audience.
And don’t stop there—diversify your sources. Pull data from social media, local news, literature, and even user-generated content. The more varied your multilingual AI training data, the better your AI will be at handling real-world scenarios.
- Leverage AI-Powered Translation Tools for Efficiency
Here’s where things get meta: use AI to train AI. AI-powered translation tools can be a lifesaver when it comes to scaling your localization efforts. Tools like neural machine translation (NMT) can handle the heavy lifting of translating large datasets, while you focus on fine-tuning the results.
But, and this is a big but, don’t rely on these tools blindly. Always have human experts review the output to ensure accuracy and cultural relevance. Because nothing screams “amateur hour” like a chatbot that sounds like it’s been Google Translated.
- Continuously Test and Refine Localized Models
Localization isn’t a one-and-done deal. It’s an ongoing process. Once your AI model is up and running, you need to test, test, and test some more. Gather feedback from real users, monitor performance metrics, and keep an eye out for any cultural faux pas or inaccuracies.
Think of it like tuning a guitar: you’ve got to keep adjusting until it sounds just right. And as languages and cultures evolve, so should your AI. That’s the beauty of language adaptation for machine learning, it’s a living, breathing process.
Why These Practices Matter?
At the end of the day, localization for AI training data isn’t just about avoiding awkward mistakes, it’s about creating AI that feels authentic, inclusive, and relatable.
By collaborating with experts, using diverse datasets, leveraging smart tools, and continuously refining your models, you’re not just building AI, you’re building trust.
And in a world where consumers are increasingly savvy about tech, that trust is worth its weight in gold.
Types of AI Training Data That Require Localization
So, you’re sold on the importance of localization for AI training data. But here’s the thing: not all data is the same. Just like you wouldn’t use a wrench to hammer a nail, you can’t approach every type of data with the same localization strategy. Let’s break it down and talk about the different kinds of data that need that special localized touch.
- Text Data: The Words Matter
Text is the bread and butter of AI training, especially for natural language processing (NLP) models. But here’s the catch: text isn’t just text. A customer review in English reads very differently from one in Japanese or Arabic.
Examples of text data that need AI dataset localization:
- Customer reviews: Imagine a review saying “This product is fire!” In the U.S., that’s a compliment. In the UK? Might sound like the product is literally on fire.
- Social media posts: Slang, hashtags, and emojis vary wildly across cultures. What’s cool in one country might be cringe in another.
- Legal documents: These need to be precise and culturally accurate. A mistranslated clause could lead to serious legal headaches.
Bottom line: language adaptation for machine learning is key to making sure your AI understands and respects the nuances of text data.
2. Speech Data: It’s Not Just What You Say, But How You Say It
Voice assistants, speech-to-text apps, and call center bots all rely on speech data. But here’s the kicker: accents, dialects, and intonation can completely change the meaning of a sentence.
Examples of speech data that need localization:
- Voice commands: “Play my workout playlist” might sound different in Texas vs. London.
- Accents and dialects: An AI trained on American English might struggle with Indian or Australian accents.
- Tone and context: Sarcasm or excitement in one language might not translate the same way in another.
Without multilingual AI training data for speech, your AI might end up sounding like it’s from another planet, or worse, failing to understand users altogether.
3. Visual Data: A Picture is Worth a Thousand (Localized) Words
Visual data is everywhere, from image recognition to video analysis. But here’s the thing: visuals are deeply cultural. A thumbs-up might mean “good job” in one place and something offensive in another.
Examples of visual data that need localization:
- Images: A wedding photo in India looks very different from one in Sweden.
- Videos: Ads, movies, and even TikTok trends need to be culturally relevant to resonate.
- Symbols and gestures: A hand gesture that’s harmless in one culture might be a major no-no in another.
Localizing visual data isn’t just about translating captions, it’s about understanding the cultural context behind the imagery.
4. Metadata and Annotations: The Unsung Heroes
Last but not least, let’s talk about metadata and annotations. These are the labels and tags that help AI make sense of data. But if they’re not localized, your AI could end up with some seriously wonky results.
Examples of metadata that need localization:
- Product tags: A “biscuit” in the U.S. is a cookie, but in the UK, it’s what Americans call a “cracker.”
- Image annotations: Labeling a “school bus” as yellow works in the U.S., but in other countries, school buses might be a different color.
- Geotags: Location-based data needs to account for regional differences in naming conventions and landmarks.
Without proper AI dataset localization for metadata, your AI might end up making some hilariously wrong assumptions.
Localization isn’t a one-size-fits-all process. Different types of data require different approaches, and getting it right is what separates a good AI model from a great one. Whether it’s text, speech, visuals, or metadata, language adaptation for machine learning ensures your AI isn’t just smart, it’s culturally savvy.
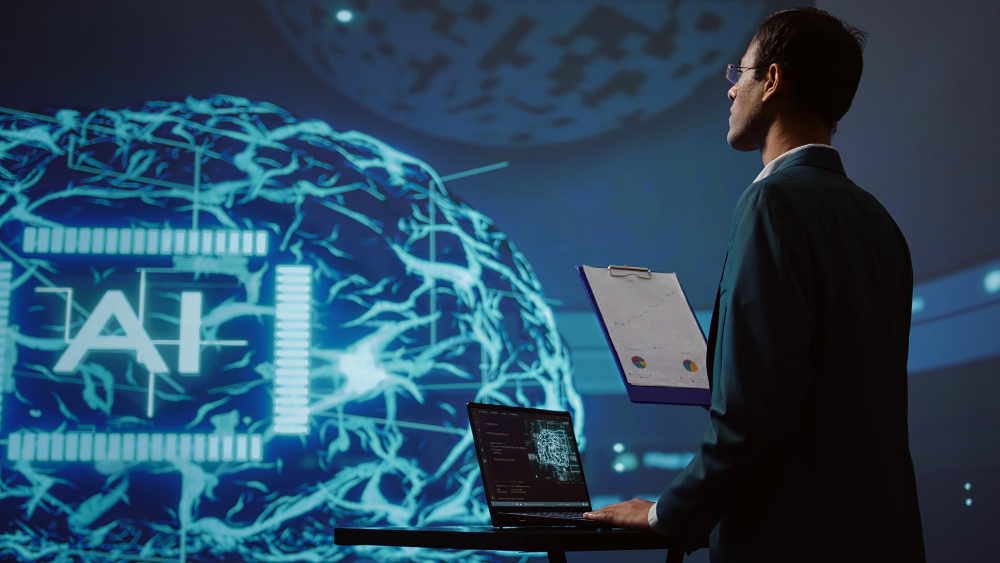
The Role of Technology in Localizing AI Training Data
Alright, so we’ve talked about the what and the why of localization for AI training data. Now, let’s geek out a bit and talk about the how. Because let’s be real: localizing data for AI isn’t just about throwing a bunch of translators at the problem. It’s about leveraging the right tech to make the process faster, smarter, and more scalable.
- Machine Translation: The First Line of Defense
When it comes to AI dataset localization, machine translation (MT) is like your trusty sidekick. Tools like Google Translate, DeepL, and custom neural machine translation (NMT) models can handle the heavy lifting of translating large volumes of text data.
But here’s the thing: MT isn’t perfect. It’s great for getting the ball rolling, but it often misses cultural nuances, idioms, and context. That’s why it’s crucial to pair MT with human review. Think of it as a tag team—AI does the bulk work, and humans fine-tune the details.
- Transcription Software: Turning Speech into Gold
Speech data is a goldmine for AI, but it’s also a beast to localize. That’s where transcription software comes in. Tools like Otter, Rev, and even custom-built solutions can convert spoken language into text, making it easier to analyze and adapt.
But here’s the most important part: transcription tools need to be trained to handle accents, dialects, and background noise. That’s where language adaptation for machine learning comes into play. By feeding these tools multilingual AI training data, you can improve their accuracy and make them more inclusive.
- Annotation Platforms: Labeling with Precision
Metadata and annotations are the unsung heroes of AI training, but manually labeling data is a time-suck. Enter annotation platforms like Labelbox, SuperAnnotate, and Prodigy. These tools help you tag, categorize, and annotate data at scale.
The best part? Many of these platforms now come with AI-powered features that can auto-label data based on patterns. But — and this is a big but — you still need human oversight to ensure those labels are culturally accurate. After all, an AI might label a “biscuit” as a cookie, but only a human can tell you if it’s the right kind of biscuit for the context.
- AI-Powered Localization: The Future is Here
Here’s where things get meta: using AI to localize AI training data. Yep, it’s a thing. AI-powered localization tools can automate parts of the process, like translating text, transcribing speech, or even suggesting culturally relevant annotations.
For example, tools like Smartling and Lokalise use AI to streamline the localization workflow, making it faster and more efficient. But the real magic happens when you combine these tools with human expertise. AI can handle the repetitive tasks, while humans focus on the creative, nuanced stuff.
The beauty of technology is that it makes localization for AI training data more scalable than ever. But here’s the catch: you can’t automate everything. Cultural nuances, context, and creativity still require a human touch.
The goal is to strike a balance, use tech to handle the heavy lifting, but always keep humans in the loop to ensure quality. After all, the last thing you want is an AI that sounds like it’s been run through Google Translate and called it a day.
The Future of Localization for AI Training Data: What’s Next?
So, what’s on the horizon for multilingual AI training data? Here are a few trends to watch:
- Increased Demand for Multilingual AI Models: As businesses expand globally, the need for AI that speaks multiple languages and dialects will only grow. Think voice assistants that can switch between Mandarin and Spanish, or chatbots that understand both formal and informal French.
- Advancements in Localization Technology: AI-powered tools are getting smarter, faster, and more accurate. We’re talking about neural networks that can handle low-resource languages, auto-annotation platforms that learn as they go, and translation tools that capture cultural nuances like never before.
- Ethical and Inclusive AI: The conversation around bias and inclusivity isn’t going away. Businesses will need to prioritize ethical localization practices, ensuring their AI models are fair, unbiased, and respectful of all cultures.
- Hyper-Personalization: The future of AI isn’t just about understanding languages, it’s about understanding people. Expect to see more AI models that adapt to individual users’ preferences, dialects, and even cultural contexts.
Your Next Step: Partner with Localizera
If there’s one takeaway from all this, it’s that localization for AI training data is a game-changer. But let’s be real: pulling it off isn’t easy. That’s where we come in.
At Localizera, we specialize in helping businesses like yours build AI models that are as global as your ambitions. From language adaptation for machine learning to multilingual AI training data, we’ve got the tools, expertise, and cultural know-how to make your AI truly world-class.
So, what are you waiting for? Whether you’re building a voice assistant, a chatbot, or a recommendation engine, we’re here to help you nail localization and take your AI to the next level.
Ready to Get Started?
Don’t let your AI get lost in translation. Partner with Localizera today and build AI systems that work for everyone, everywhere. Let’s make your AI not just smart, but globally brilliant.
Contact us now to learn more about our AI dataset localization services and start your journey toward truly inclusive AI. The future of AI is multilingual, let’s build it together.